As the overall data landscape somewhat sobers up from the excesses of previous years, we see the balance of power between data organizations and their business and finance counterparts evolve.
At the same time the massive evolution of data technology opens up new possibilities. Will they materialize in quantifiable business value? Time will tell but what is certain is that success with data will need to play on all fronts: business value first but also organizational effectiveness and scalability of infrastructures.
Business value from data at an affordable long-term cost, this is probably the common denominator of all the themes we outline for the year.
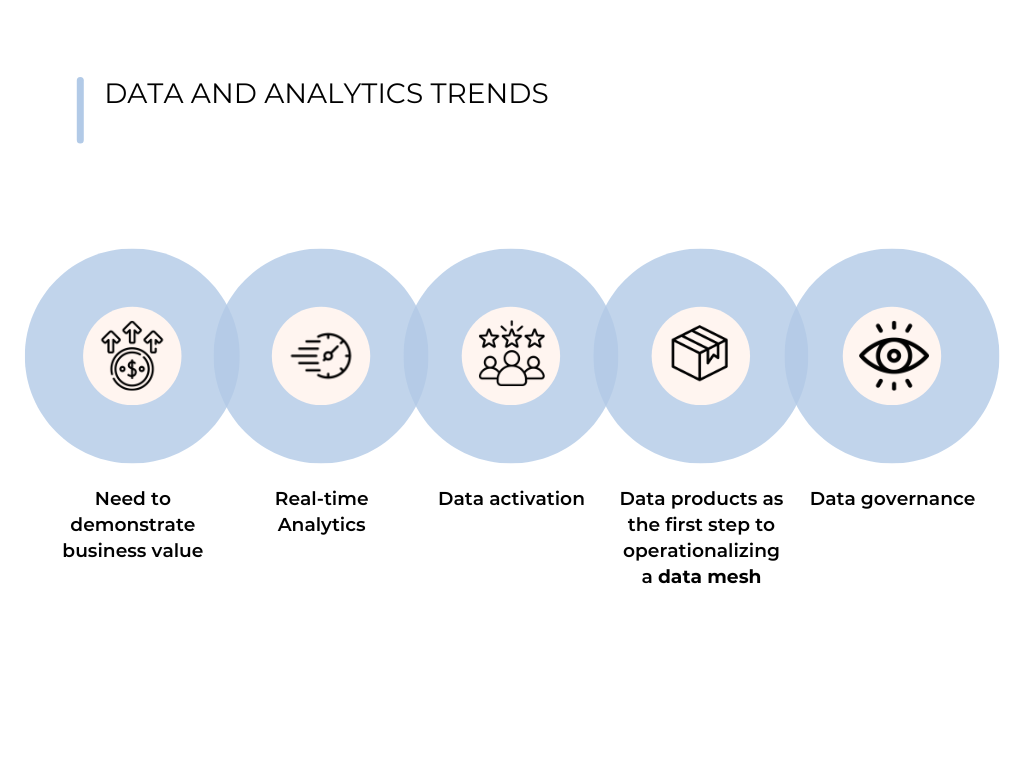
2023 Five Top Trends
1. Need to demonstrate value
What is probably the biggest challenge of Chief Data Officers these days is the disconnect between their need to demonstrate business value to their business executives and the complexity of all the technical aspects of data that are brought back to them by their own teams.
Up till very recently, it was obvious to everyone that data was the new oil and that any investment in data would bring massive pay-backs.
In a tighter business context, the massive investments made in data technology are getting pressured to deliver tangible business returns. The move to the cloud which was seen as the panacea to simplify data infrastructure is coming with hefty recurring bills and many promising projects are either struggling to get into production or are generating largely intangible value.
In that context, the good old recipe of demonstrating business ROI is getting back to the top.
I find this trend overall very positive as keeping the business on board is certainly critical for the continuous success of data teams. At the same time, the risk is that as this trend develops, core investments in data infrastructure might become harder to justify as they don’t bring immediate business returns.
Strong CDOs will certainly be able to defend data infrastructure and governance initiatives on the basis of economies of scale and non-linear data exploitation costs, yet this remains a point of attention.
2. Real-time analytics
The appetite for real-time analytics continues to be high. The notion of human-time data-driven decision making is very appealing in many business contexts. It is when the customer is in front of you or interacting through your e-commerce or customer engagement channels that you can have the maximum impact. Smart grids, mobility, logistics, fraud detection, cyber-attacks are some of the other most prevalent use cases that are begging for real-time solutions.
While the (not so) modern data stack is still very much centered on batch processing, we are starting to see alignment on the streaming technology end-to-end data stack.
I have been delighted to see that the technology stack on which we have been working for the last four years (Apache Kafka + Flink) is now being embraced by the market as best practice (cfr the recent announcement of the acquisition of Imerok by Confluent).
Real-time analytics will not replace batches everywhere, which means that the technologies will co-exist for the foreseeable future and that has implications in terms of standardization and governance.
3. Data activation
Data activation sometimes referred to as operational analytics is the ability to exploit analytical insight in operational processes.
One business area which has been ahead of the curve in this process is digital marketing with the development of the Customer Data Platform (CDP) market. The expanding possibilities provided by AI models make the operationalization of these models more and more appealing for improved customer interactions.
What is interesting to witness is the whole narrative provided by reverse ETL vendors around the modern data stack. It is supposedly becoming so easy to activate data around that technology stack that it will drive to obsolescence specialized solutions like CDPs or other domain-specific SaaS offerings.
This is a very bold claim and I am not convinced that this prediction will materialize. The real question I have in this space is whether streaming technology is not a better technology candidate to make data activation effective across an enterprise. It feels to me that in a data activation context, event-based, real-time approaches offer more business possibilities than warehouse-centric approaches.
4. Data products as the first step to operationalizing a data mesh
The concept of data mesh is very exciting as it is based on strong top-down logic: if a centralized approach to data is creating too much of a bottleneck, the way forward has to be a decentralized yet federated approach. It is only when you start imagining what it could mean to your organization that you get faced with the massive change management challenges.
The implications go far beyond the technology and if you want an approach that works in practice, you need to take into consideration all the process and organizational aspects. In other words, you need to design an operational model for the data mesh in your organization.
One of the core concepts of data mesh, data products, is gaining more and more traction as a first practical step to moving towards a data mesh.
The simplest way to explain this success is that a data product is at its heart just a data set optimized for consumption. This means that you are not starting from scratch as most enterprises already have many data sets of reasonable quality.
The value of the approach comes back to the first trend that we have explored: the data needs to be packaged to maximize the enterprise’s ability to create business value from it and to monitor that value through time.
Data governance continues to be a priority in all the organizations we talk to. The regulatory aspects like the conformance to GDPR regulation make governance investments a must in any case. Beyond that, the increasing complexity of the organization and technology aspects make additional aspects of data governance like data quality programs or standardization efforts very desirable.
Governance investments can sometimes be tricky as the narratives oscillate between a necessary evil and an absolute necessity on the path to business value. CDOs can have a hard time selling the value of data governance programs to business executives eager to get immediate business benefits.
Given the overall business context, the governance function needs to embrace more and more the path to demonstrating business value. It also has a big role to play in designing effective operationalized approaches that can keep the long-term cost of data exploitation affordable.
A data value management strategy focuses on enabling data-driven business use cases but also on identifying data that brings the most value and on ensuring its effective maintenance. The sustainable creation of business value is a great way of showing the value of data governance to the business.
The data and analytics landscape continues to be very hard to read with more narratives flying around than the real needs for effective solutions. Nobody is questioning the massive business value that can be generated from intelligent use of data yet business accountability is back in full force.
The need for efficient data infrastructure and a stronger alignment to business value generation seems to remain a central theme across all the scenarios that we can imagine for the near future.
Digazu for Seamless Integration
Digazu is a software solution that packages the required technologies for stream integration in a low-code approach. With Digazu, non-experts can easily create real-time data pipelines and integrate them with existing BI tools without impacting business processes that do not require real-time data.
With Digazu, not only will you be able to maintain well-functioning BI tools as well as BI chains, hence minimising disruption but you will also be able to upgrade your data integration with real-time capabilities while ensuring seamless integration with your current systems and BI environments.
Real-time data integration for business intelligence presents many challenges. However, with the right approach, these challenges can be overcome. It is essential to select the right technology and infrastructure to manage and analyse the data effectively.